
Summary
Generative AI has rapidly transformed everything in the technology sector. When Andrew Lee started work on Shortwave he was focused on making email more productive. When AI started gaining adoption he realized that he had even more potential for a transformative experience. In this episode he shares the technical challenges that he and his team have overcome in integrating AI into their product, as well as the benefits and features that it provides to their customers.
Announcements
Hello and welcome to the Data Engineering Podcast, the show about modern data management
Dagster offers a new approach to building and running data platforms and data pipelines. It is an open-source, cloud-native orchestrator for the whole development lifecycle, with integrated lineage and observability, a declarative programming model, and best-in-class testability. Your team can get up and running in minutes thanks to Dagster Cloud, an enterprise-class hosted solution that offers serverless and hybrid deployments, enhanced security, and on-demand ephemeral test deployments. Go to dataengineeringpodcast.com/dagster today to get started. Your first 30 days are free!
Data lakes are notoriously complex. For data engineers who battle to build and scale high quality data workflows on the data lake, Starburst powers petabyte-scale SQL analytics fast, at a fraction of the cost of traditional methods, so that you can meet all your data needs ranging from AI to data applications to complete analytics. Trusted by teams of all sizes, including Comcast and Doordash, Starburst is a data lake analytics platform that delivers the adaptability and flexibility a lakehouse ecosystem promises. And Starburst does all of this on an open architecture with first-class support for Apache Iceberg, Delta Lake and Hudi, so you always maintain ownership of your data. Want to see Starburst in action? Go to dataengineeringpodcast.com/starburst and get $500 in credits to try Starburst Galaxy today, the easiest and fastest way to get started using Trino.
Your host is Tobias Macey and today I'm interviewing Andrew Lee about his work on Shortwave, an AI powered email client
Interview
Introduction
How did you get involved in the area of data management?
Can you describe what Shortwave is and the story behind it?
What is the core problem that you are addressing with Shortwave?
Email has been a central part of communication and business productivity for decades now. What are the overall themes that continue to be problematic?
What are the strengths that email maintains as a protocol and ecosystem?
From a product perspective, what are the data challenges that are posed by email?
Can you describe how you have architected the Shortwave platform?
How have the design and goals of the product changed since you started it?
What are the ways that the advent and evolution of language models have influenced your product roadmap?
How do you manage the personalization of the AI functionality in your system for each user/team?
For users and teams who are using Shortwave, how does it change their workflow and communication patterns?
Can you describe how I would use Shortwave for managing the workflow of evaluating, planning, and promoting my podcast episodes?
What are the most interesting, innovative, or unexpected ways that you have seen Shortwave used?
What are the most interesting, unexpected, or challenging lessons that you have learned while working on Shortwave?
When is Shortwave the wrong choice?
What do you have planned for the future of Shortwave?
Contact Info
LinkedIn
Blog
Parting Question
From your perspective, what is the biggest gap in the tooling or technology for data management today?
Closing Announcements
Thank you for listening! Don't forget to check out our other shows. Podcast.__init__ covers the Python language, its community, and the innovative ways it is being used. The Machine Learning Podcast helps you go from idea to production with machine learning.
Visit the site to subscribe to the show, sign up for the mailing list, and read the show notes.
If you've learned something or tried out a project from the show then tell us about it! Email hosts@dataengineeringpodcast.com) with your story.
Links
Shortwave
Firebase
Google Inbox
Hey
Ezra Klein Hey Article
Superhuman
Pinecone
Podcast Episode
Elastic
Hybrid Search
Semantic Search
Mistral
GPT 3.5
IMAP
The intro and outro music is from The Hug by The Freak Fandango Orchestra / CC BY-SASponsored By:Starburst: 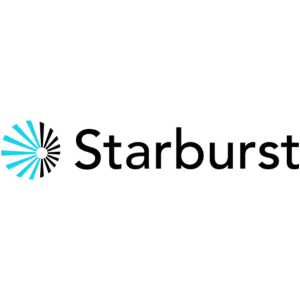
This episode is brought to you by Starburst - a data lake analytics platform for data engineers who are battling to build and scale high quality data pipelines on the data lake. Powered by Trino, Starburst runs petabyte-scale SQL analytics fast at a fraction of the cost of traditional methods, helping you meet all your data needs ranging from AI/ML workloads to data applications to complete analytics.
Trusted by the teams at Comcast and Doordash, Starburst delivers the adaptability and flexibility a lakehouse ecosystem promises, while providing a single point of access for your data and all your data governance allowing you to discover, transform, govern, and secure all in one place. Starburst does all of this on an open architecture with first-class support for Apache Iceberg, Delta Lake and Hudi, so you always maintain ownership of your data. Want to see Starburst in action? Try Starburst Galaxy today, the easiest and fastest way to get started using Trino, and get $500 of credits free. [dataengineeringpodcast.com/starburst](https://www.dataengineeringpodcast.com/starburst)Dagster: 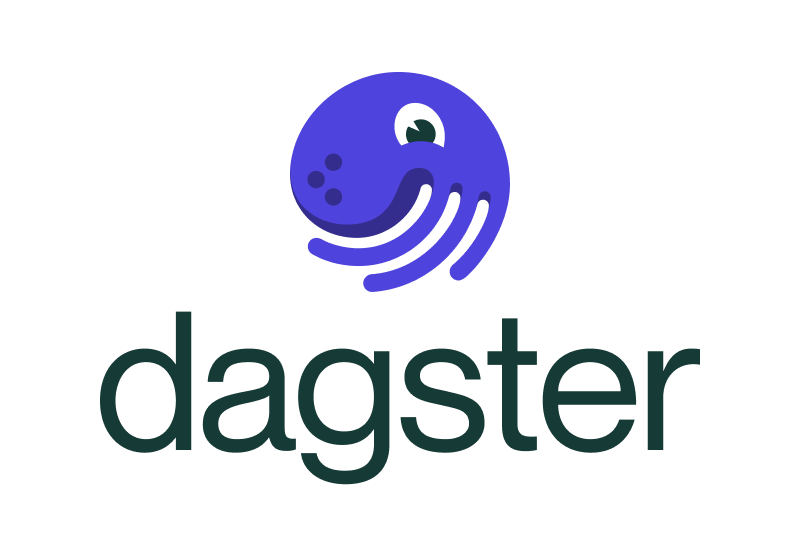
Data teams are tasked with helping organizations deliver on the premise of data, and with ML and AI maturing rapidly, expectations have never been this high. However data engineers are challenged by both technical complexity and organizational complexity, with heterogeneous technologies to adopt, multiple data disciplines converging, legacy systems to support, and costs to manage.
Dagster is an open-source orchestration solution that helps data teams reign in this complexity and build data platforms that provide unparalleled observability, and testability, all while fostering collaboration across the enterprise. With enterprise-grade hosting on Dagster Cloud, you gain even more capabilities, adding cost management, security, and CI support to further boost your teams' productivity. Go to [dagster.io](https://dagster.io/lp/dagster-cloud-trial?source=data-eng-podcast) today to get your first 30 days free!Support Data Engineering Podcast
Apr 21
53 min

Summary
Databases come in a variety of formats for different use cases. The default association with the term "database" is relational engines, but non-relational engines are also used quite widely. In this episode Oren Eini, CEO and creator of RavenDB, explores the nuances of relational vs. non-relational engines, and the strategies for designing a non-relational database.
Announcements
Hello and welcome to the Data Engineering Podcast, the show about modern data management
This episode is brought to you by Datafold – a testing automation platform for data engineers that prevents data quality issues from entering every part of your data workflow, from migration to dbt deployment. Datafold has recently launched data replication testing, providing ongoing validation for source-to-target replication. Leverage Datafold's fast cross-database data diffing and Monitoring to test your replication pipelines automatically and continuously. Validate consistency between source and target at any scale, and receive alerts about any discrepancies. Learn more about Datafold by visiting dataengineeringpodcast.com/datafold.
Dagster offers a new approach to building and running data platforms and data pipelines. It is an open-source, cloud-native orchestrator for the whole development lifecycle, with integrated lineage and observability, a declarative programming model, and best-in-class testability. Your team can get up and running in minutes thanks to Dagster Cloud, an enterprise-class hosted solution that offers serverless and hybrid deployments, enhanced security, and on-demand ephemeral test deployments. Go to dataengineeringpodcast.com/dagster today to get started. Your first 30 days are free!
Data lakes are notoriously complex. For data engineers who battle to build and scale high quality data workflows on the data lake, Starburst powers petabyte-scale SQL analytics fast, at a fraction of the cost of traditional methods, so that you can meet all your data needs ranging from AI to data applications to complete analytics. Trusted by teams of all sizes, including Comcast and Doordash, Starburst is a data lake analytics platform that delivers the adaptability and flexibility a lakehouse ecosystem promises. And Starburst does all of this on an open architecture with first-class support for Apache Iceberg, Delta Lake and Hudi, so you always maintain ownership of your data. Want to see Starburst in action? Go to dataengineeringpodcast.com/starburst and get $500 in credits to try Starburst Galaxy today, the easiest and fastest way to get started using Trino.
Your host is Tobias Macey and today I'm interviewing Oren Eini about the work of designing and building a NoSQL database engine
Interview
Introduction
How did you get involved in the area of data management?
Can you describe what constitutes a NoSQL database?
How have the requirements and applications of NoSQL engines changed since they first became popular ~15 years ago?
What are the factors that convince teams to use a NoSQL vs. SQL database?
NoSQL is a generalized term that encompasses a number of different data models. How does the underlying representation (e.g. document, K/V, graph) change that calculus?
How have the evolution in data formats (e.g. N-dimensional vectors, point clouds, etc.) changed the landscape for NoSQL engines?
When designing and building a database, what are the initial set of questions that need to be answered?
How many "core capabilities" can you reasonably design around before they conflict with each other?
How have you approached the evolution of RavenDB as you add new capabilities and mature the project?
What are some of the early decisions that had to be unwound to enable new capabilities?
If you were to start from scratch today, what database would you build?
What are the most interesting, innovative, or unexpected ways that you have seen RavenDB/NoSQL databases used?
What are the most interesting, unexpected, or challenging lessons that you have learned while working on RavenDB?
When is a NoSQL database/RavenDB the wrong choice?
What do you have planned for the future of RavenDB?
Contact Info
Blog
LinkedIn
Parting Question
From your perspective, what is the biggest gap in the tooling or technology for data management today?
Closing Announcements
Thank you for listening! Don't forget to check out our other shows. Podcast.__init__ covers the Python language, its community, and the innovative ways it is being used. The Machine Learning Podcast helps you go from idea to production with machine learning.
Visit the site to subscribe to the show, sign up for the mailing list, and read the show notes.
If you've learned something or tried out a project from the show then tell us about it! Email hosts@dataengineeringpodcast.com) with your story.
Links
RavenDB
RSS
Object Relational Mapper (ORM)
Relational Database
NoSQL
CouchDB
Navigational Database
MongoDB
Redis
Neo4J
Cassandra
Column-Family
SQLite
LevelDB
Firebird DB
fsync
Esent DB?
KNN == K-Nearest Neighbors
RocksDB
C# Language
ASP.NET
QUIC
Dynamo Paper
Database Internals book (affiliate link)
Designing Data Intensive Applications book (affiliate link)
The intro and outro music is from The Hug by The Freak Fandango Orchestra / CC BY-SASponsored By:Starburst: 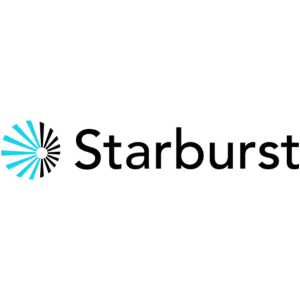
This episode is brought to you by Starburst - a data lake analytics platform for data engineers who are battling to build and scale high quality data pipelines on the data lake. Powered by Trino, Starburst runs petabyte-scale SQL analytics fast at a fraction of the cost of traditional methods, helping you meet all your data needs ranging from AI/ML workloads to data applications to complete analytics.
Trusted by the teams at Comcast and Doordash, Starburst delivers the adaptability and flexibility a lakehouse ecosystem promises, while providing a single point of access for your data and all your data governance allowing you to discover, transform, govern, and secure all in one place. Starburst does all of this on an open architecture with first-class support for Apache Iceberg, Delta Lake and Hudi, so you always maintain ownership of your data. Want to see Starburst in action? Try Starburst Galaxy today, the easiest and fastest way to get started using Trino, and get $500 of credits free. [dataengineeringpodcast.com/starburst](https://www.dataengineeringpodcast.com/starburst)Datafold: 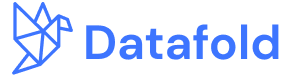
This episode is brought to you by Datafold – a testing automation platform for data engineers that prevents data quality issues from entering every part of your data workflow, from migration to dbt deployment. Datafold has recently launched data replication testing, providing ongoing validation for source-to-target replication. Leverage Datafold's fast cross-database data diffing and Monitoring to test your replication pipelines automatically and continuously. Validate consistency between source and target at any scale, and receive alerts about any discrepancies. Learn more about Datafold by visiting https://get.datafold.com/replication-de-podcast.Dagster: 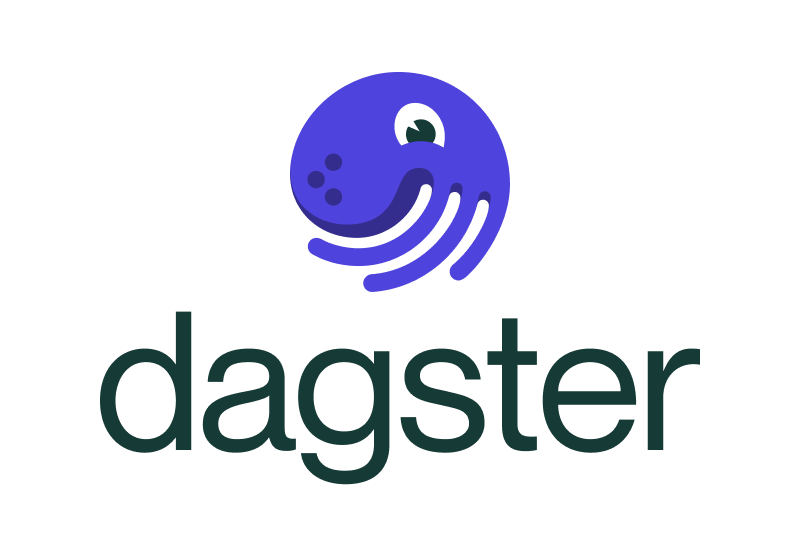
Data teams are tasked with helping organizations deliver on the premise of data, and with ML and AI maturing rapidly, expectations have never been this high. However data engineers are challenged by both technical complexity and organizational complexity, with heterogeneous technologies to adopt, multiple data disciplines converging, legacy systems to support, and costs to manage.
Dagster is an open-source orchestration solution that helps data teams reign in this complexity and build data platforms that provide unparalleled observability, and testability, all while fostering collaboration across the enterprise. With enterprise-grade hosting on Dagster Cloud, you gain even more capabilities, adding cost management, security, and CI support to further boost your teams' productivity. Go to [dagster.io](https://dagster.io/lp/dagster-cloud-trial?source=data-eng-podcast) today to get your first 30 days free!Support Data Engineering Podcast
Apr 14
1 hr 16 min

Summary
Maintaining a single source of truth for your data is the biggest challenge in data engineering. Different roles and tasks in the business need their own ways to access and analyze the data in the organization. In order to enable this use case, while maintaining a single point of access, the semantic layer has evolved as a technological solution to the problem. In this episode Artyom Keydunov, creator of Cube, discusses the evolution and applications of the semantic layer as a component of your data platform, and how Cube provides speed and cost optimization for your data consumers.
Announcements
Hello and welcome to the Data Engineering Podcast, the show about modern data management
This episode is brought to you by Datafold – a testing automation platform for data engineers that prevents data quality issues from entering every part of your data workflow, from migration to dbt deployment. Datafold has recently launched data replication testing, providing ongoing validation for source-to-target replication. Leverage Datafold's fast cross-database data diffing and Monitoring to test your replication pipelines automatically and continuously. Validate consistency between source and target at any scale, and receive alerts about any discrepancies. Learn more about Datafold by visiting dataengineeringpodcast.com/datafold.
Dagster offers a new approach to building and running data platforms and data pipelines. It is an open-source, cloud-native orchestrator for the whole development lifecycle, with integrated lineage and observability, a declarative programming model, and best-in-class testability. Your team can get up and running in minutes thanks to Dagster Cloud, an enterprise-class hosted solution that offers serverless and hybrid deployments, enhanced security, and on-demand ephemeral test deployments. Go to dataengineeringpodcast.com/dagster today to get started. Your first 30 days are free!
Data lakes are notoriously complex. For data engineers who battle to build and scale high quality data workflows on the data lake, Starburst powers petabyte-scale SQL analytics fast, at a fraction of the cost of traditional methods, so that you can meet all your data needs ranging from AI to data applications to complete analytics. Trusted by teams of all sizes, including Comcast and Doordash, Starburst is a data lake analytics platform that delivers the adaptability and flexibility a lakehouse ecosystem promises. And Starburst does all of this on an open architecture with first-class support for Apache Iceberg, Delta Lake and Hudi, so you always maintain ownership of your data. Want to see Starburst in action? Go to dataengineeringpodcast.com/starburst and get $500 in credits to try Starburst Galaxy today, the easiest and fastest way to get started using Trino.
Your host is Tobias Macey and today I'm interviewing Artyom Keydunov about the role of the semantic layer in your data platform
Interview
Introduction
How did you get involved in the area of data management?
Can you start by outlining the technical elements of what it means to have a "semantic layer"?
In the past couple of years there was a rapid hype cycle around the "metrics layer" and "headless BI", which has largely faded. Can you give your assessment of the current state of the industry around the adoption/implementation of these concepts?
What are the benefits of having a discrete service that offers the business metrics/semantic mappings as opposed to implementing those concepts as part of a more general system? (e.g. dbt, BI, warehouse marts, etc.)
At what point does it become necessary/beneficial for a team to adopt such a service?
What are the challenges involved in retrofitting a semantic layer into a production data system?
evolution of requirements/usage patterns
technical complexities/performance and cost optimization
What are the most interesting, innovative, or unexpected ways that you have seen Cube used?
What are the most interesting, unexpected, or challenging lessons that you have learned while working on Cube?
When is Cube/a semantic layer the wrong choice?
What do you have planned for the future of Cube?
Contact Info
LinkedIn
keydunov on GitHub
Parting Question
From your perspective, what is the biggest gap in the tooling or technology for data management today?
Closing Announcements
Thank you for listening! Don't forget to check out our other shows. Podcast.__init__ covers the Python language, its community, and the innovative ways it is being used. The Machine Learning Podcast helps you go from idea to production with machine learning.
Visit the site to subscribe to the show, sign up for the mailing list, and read the show notes.
If you've learned something or tried out a project from the show then tell us about it! Email hosts@dataengineeringpodcast.com) with your story.
Links
Cube
Semantic Layer
Business Objects
Tableau
Looker
Podcast Episode
Mode
Thoughtspot
LightDash
Podcast Episode
Embedded Analytics
Dimensional Modeling
Clickhouse
Podcast Episode
Druid
BigQuery
Starburst
Pinot
Snowflake
Podcast Episode
Arrow Datafusion
Metabase
Podcast Episode
Superset
Alation
Collibra
Podcast Episode
Atlan
Podcast Episode
The intro and outro music is from The Hug by The Freak Fandango Orchestra / CC BY-SASponsored By:Starburst: 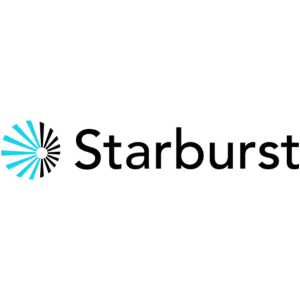
This episode is brought to you by Starburst - a data lake analytics platform for data engineers who are battling to build and scale high quality data pipelines on the data lake. Powered by Trino, Starburst runs petabyte-scale SQL analytics fast at a fraction of the cost of traditional methods, helping you meet all your data needs ranging from AI/ML workloads to data applications to complete analytics.
Trusted by the teams at Comcast and Doordash, Starburst delivers the adaptability and flexibility a lakehouse ecosystem promises, while providing a single point of access for your data and all your data governance allowing you to discover, transform, govern, and secure all in one place. Starburst does all of this on an open architecture with first-class support for Apache Iceberg, Delta Lake and Hudi, so you always maintain ownership of your data. Want to see Starburst in action? Try Starburst Galaxy today, the easiest and fastest way to get started using Trino, and get $500 of credits free. [dataengineeringpodcast.com/starburst](https://www.dataengineeringpodcast.com/starburst)Datafold: 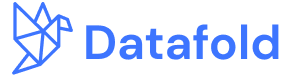
This episode is brought to you by Datafold – a testing automation platform for data engineers that prevents data quality issues from entering every part of your data workflow, from migration to dbt deployment. Datafold has recently launched data replication testing, providing ongoing validation for source-to-target replication. Leverage Datafold's fast cross-database data diffing and Monitoring to test your replication pipelines automatically and continuously. Validate consistency between source and target at any scale, and receive alerts about any discrepancies. Learn more about Datafold by visiting https://get.datafold.com/replication-de-podcast.Dagster: 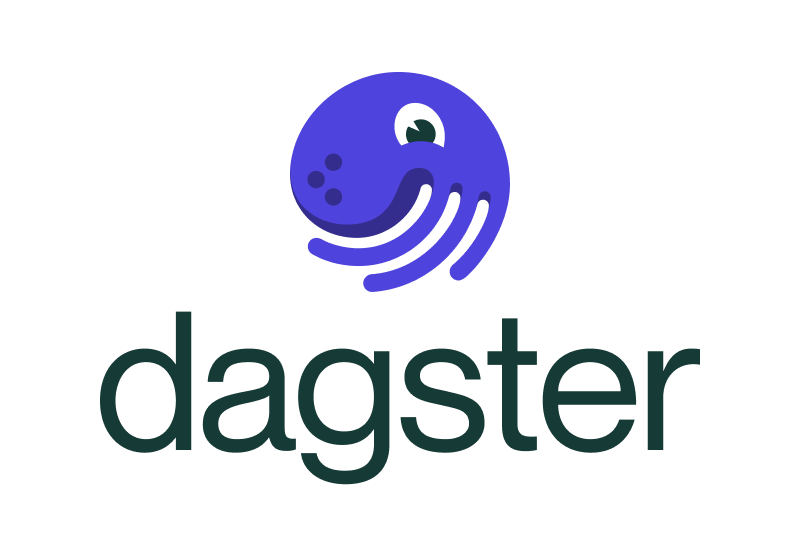
Data teams are tasked with helping organizations deliver on the premise of data, and with ML and AI maturing rapidly, expectations have never been this high. However data engineers are challenged by both technical complexity and organizational complexity, with heterogeneous technologies to adopt, multiple data disciplines converging, legacy systems to support, and costs to manage.
Dagster is an open-source orchestration solution that helps data teams reign in this complexity and build data platforms that provide unparalleled observability, and testability, all while fostering collaboration across the enterprise. With enterprise-grade hosting on Dagster Cloud, you gain even more capabilities, adding cost management, security, and CI support to further boost your teams' productivity. Go to [dagster.io](https://dagster.io/lp/dagster-cloud-trial?source=data-eng-podcast) today to get your first 30 days free!Support Data Engineering Podcast
Apr 7
56 min

Summary
Working with data is a complicated process, with numerous chances for something to go wrong. Identifying and accounting for those errors is a critical piece of building trust in the organization that your data is accurate and up to date. While there are numerous products available to provide that visibility, they all have different technologies and workflows that they focus on. To bring observability to dbt projects the team at Elementary embedded themselves into the workflow. In this episode Maayan Salom explores the approach that she has taken to bring observability, enhanced testing capabilities, and anomaly detection into every step of the dbt developer experience.
Announcements
Hello and welcome to the Data Engineering Podcast, the show about modern data management
Data lakes are notoriously complex. For data engineers who battle to build and scale high quality data workflows on the data lake, Starburst powers petabyte-scale SQL analytics fast, at a fraction of the cost of traditional methods, so that you can meet all your data needs ranging from AI to data applications to complete analytics. Trusted by teams of all sizes, including Comcast and Doordash, Starburst is a data lake analytics platform that delivers the adaptability and flexibility a lakehouse ecosystem promises. And Starburst does all of this on an open architecture with first-class support for Apache Iceberg, Delta Lake and Hudi, so you always maintain ownership of your data. Want to see Starburst in action? Go to dataengineeringpodcast.com/starburst and get $500 in credits to try Starburst Galaxy today, the easiest and fastest way to get started using Trino.
Dagster offers a new approach to building and running data platforms and data pipelines. It is an open-source, cloud-native orchestrator for the whole development lifecycle, with integrated lineage and observability, a declarative programming model, and best-in-class testability. Your team can get up and running in minutes thanks to Dagster Cloud, an enterprise-class hosted solution that offers serverless and hybrid deployments, enhanced security, and on-demand ephemeral test deployments. Go to dataengineeringpodcast.com/dagster today to get started. Your first 30 days are free!
This episode is brought to you by Datafold – a testing automation platform for data engineers that prevents data quality issues from entering every part of your data workflow, from migration to dbt deployment. Datafold has recently launched data replication testing, providing ongoing validation for source-to-target replication. Leverage Datafold's fast cross-database data diffing and Monitoring to test your replication pipelines automatically and continuously. Validate consistency between source and target at any scale, and receive alerts about any discrepancies. Learn more about Datafold by visiting dataengineeringpodcast.com/datafold.
Your host is Tobias Macey and today I'm interviewing Maayan Salom about how to incorporate observability into a dbt-oriented workflow and how Elementary can help
Interview
Introduction
How did you get involved in the area of data management?
Can you start by outlining what elements of observability are most relevant for dbt projects?
What are some of the common ad-hoc/DIY methods that teams develop to acquire those insights?
What are the challenges/shortcomings associated with those approaches?
Over the past ~3 years there were numerous data observability systems/products created. What are some of the ways that the specifics of dbt workflows are not covered by those generalized tools?
What are the insights that can be more easily generated by embedding into the dbt toolchain and development cycle?
Can you describe what Elementary is and how it is designed to enhance the development and maintenance work in dbt projects?
How is Elementary designed/implemented?
How have the scope and goals of the project changed since you started working on it?
What are the engineering challenges/frustrations that you have dealt with in the creation and evolution of Elementary?
Can you talk us through the setup and workflow for teams adopting Elementary in their dbt projects?
How does the incorporation of Elementary change the development habits of the teams who are using it?
What are the most interesting, innovative, or unexpected ways that you have seen Elementary used?
What are the most interesting, unexpected, or challenging lessons that you have learned while working on Elementary?
When is Elementary the wrong choice?
What do you have planned for the future of Elementary?
Contact Info
LinkedIn
Parting Question
From your perspective, what is the biggest gap in the tooling or technology for data management today?
Closing Announcements
Thank you for listening! Don't forget to check out our other shows. Podcast.__init__ covers the Python language, its community, and the innovative ways it is being used. The Machine Learning Podcast helps you go from idea to production with machine learning.
Visit the site to subscribe to the show, sign up for the mailing list, and read the show notes.
If you've learned something or tried out a project from the show then tell us about it! Email hosts@dataengineeringpodcast.com) with your story.
Links
Elementary
Data Observability
dbt
Datadog
pre-commit
dbt packages
SQLMesh
Malloy
SDF
The intro and outro music is from The Hug by The Freak Fandango Orchestra / CC BY-SASponsored By:Starburst: 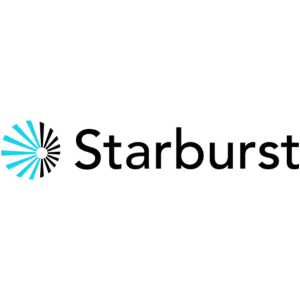
This episode is brought to you by Starburst - a data lake analytics platform for data engineers who are battling to build and scale high quality data pipelines on the data lake. Powered by Trino, Starburst runs petabyte-scale SQL analytics fast at a fraction of the cost of traditional methods, helping you meet all your data needs ranging from AI/ML workloads to data applications to complete analytics.
Trusted by the teams at Comcast and Doordash, Starburst delivers the adaptability and flexibility a lakehouse ecosystem promises, while providing a single point of access for your data and all your data governance allowing you to discover, transform, govern, and secure all in one place. Starburst does all of this on an open architecture with first-class support for Apache Iceberg, Delta Lake and Hudi, so you always maintain ownership of your data. Want to see Starburst in action? Try Starburst Galaxy today, the easiest and fastest way to get started using Trino, and get $500 of credits free. [dataengineeringpodcast.com/starburst](https://www.dataengineeringpodcast.com/starburst)Datafold: 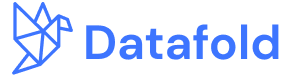
This episode is brought to you by Datafold – a testing automation platform for data engineers that prevents data quality issues from entering every part of your data workflow, from migration to dbt deployment. Datafold has recently launched data replication testing, providing ongoing validation for source-to-target replication. Leverage Datafold's fast cross-database data diffing and Monitoring to test your replication pipelines automatically and continuously. Validate consistency between source and target at any scale, and receive alerts about any discrepancies. Learn more about Datafold by visiting https://get.datafold.com/replication-de-podcast.Dagster: 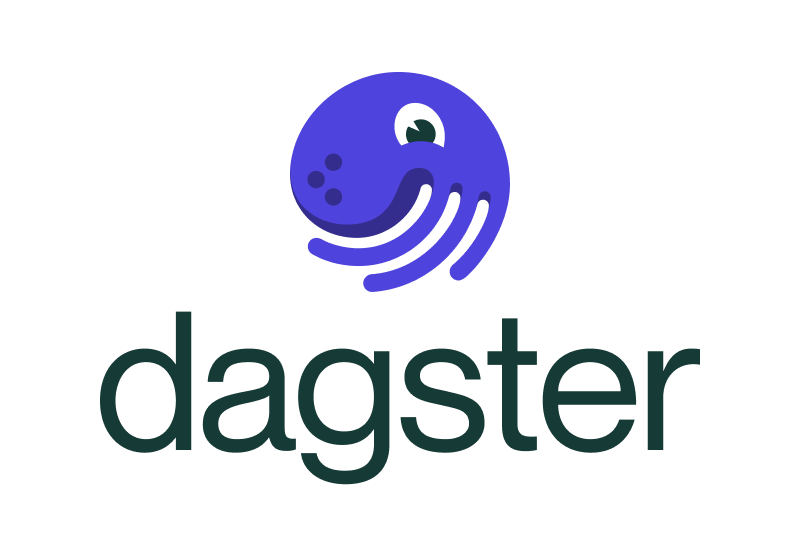
Data teams are tasked with helping organizations deliver on the premise of data, and with ML and AI maturing rapidly, expectations have never been this high. However data engineers are challenged by both technical complexity and organizational complexity, with heterogeneous technologies to adopt, multiple data disciplines converging, legacy systems to support, and costs to manage.
Dagster is an open-source orchestration solution that helps data teams reign in this complexity and build data platforms that provide unparalleled observability, and testability, all while fostering collaboration across the enterprise. With enterprise-grade hosting on Dagster Cloud, you gain even more capabilities, adding cost management, security, and CI support to further boost your teams' productivity. Go to [dagster.io](https://dagster.io/lp/dagster-cloud-trial?source=data-eng-podcast) today to get your first 30 days free!Support Data Engineering Podcast
Mar 31
50 min

Summary
A core differentiator of Dagster in the ecosystem of data orchestration is their focus on software defined assets as a means of building declarative workflows. With their launch of Dagster+ as the redesigned commercial companion to the open source project they are investing in that capability with a suite of new features. In this episode Pete Hunt, CEO of Dagster labs, outlines these new capabilities, how they reduce the burden on data teams, and the increased collaboration that they enable across teams and business units.
Announcements
Hello and welcome to the Data Engineering Podcast, the show about modern data management
Dagster offers a new approach to building and running data platforms and data pipelines. It is an open-source, cloud-native orchestrator for the whole development lifecycle, with integrated lineage and observability, a declarative programming model, and best-in-class testability. Your team can get up and running in minutes thanks to Dagster Cloud, an enterprise-class hosted solution that offers serverless and hybrid deployments, enhanced security, and on-demand ephemeral test deployments. Go to dataengineeringpodcast.com/dagster today to get started. Your first 30 days are free!
Data lakes are notoriously complex. For data engineers who battle to build and scale high quality data workflows on the data lake, Starburst powers petabyte-scale SQL analytics fast, at a fraction of the cost of traditional methods, so that you can meet all your data needs ranging from AI to data applications to complete analytics. Trusted by teams of all sizes, including Comcast and Doordash, Starburst is a data lake analytics platform that delivers the adaptability and flexibility a lakehouse ecosystem promises. And Starburst does all of this on an open architecture with first-class support for Apache Iceberg, Delta Lake and Hudi, so you always maintain ownership of your data. Want to see Starburst in action? Go to dataengineeringpodcast.com/starburst and get $500 in credits to try Starburst Galaxy today, the easiest and fastest way to get started using Trino.
Your host is Tobias Macey and today I'm interviewing Pete Hunt about how the launch of Dagster+ will level up your data platform and orchestrate across language platforms
Interview
Introduction
How did you get involved in the area of data management?
Can you describe what the focus of Dagster+ is and the story behind it?
What problems are you trying to solve with Dagster+?
What are the notable enhancements beyond the Dagster Core project that this updated platform provides?
How is it different from the current Dagster Cloud product?
In the launch announcement you tease new capabilities that would be great to explore in turns:
Make data a team sport, enabling data teams across the organization
Deliver reliable, high quality data the organization can trust
Observe and manage data platform costs
Master the heterogeneous collection of technologies—both traditional and Modern Data Stack
What are the business/product goals that you are focused on improving with the launch of Dagster+
What are the most interesting, innovative, or unexpected ways that you have seen Dagster used?
What are the most interesting, unexpected, or challenging lessons that you have learned while working on the design and launch of Dagster+?
When is Dagster+ the wrong choice?
What do you have planned for the future of Dagster/Dagster Cloud/Dagster+?
Contact Info
Twitter
LinkedIn
Parting Question
From your perspective, what is the biggest gap in the tooling or technology for data management today?
Closing Announcements
Thank you for listening! Don't forget to check out our other shows. Podcast.__init__ covers the Python language, its community, and the innovative ways it is being used. The Machine Learning Podcast helps you go from idea to production with machine learning.
Visit the site to subscribe to the show, sign up for the mailing list, and read the show notes.
If you've learned something or tried out a project from the show then tell us about it! Email hosts@dataengineeringpodcast.com) with your story.
Links
Dagster
Podcast Episode
Dagster+ Launch Event
Hadoop
MapReduce
Pydantic
Software Defined Assets
Dagster Insights
Dagster Pipes
Conway's Law
Data Mesh
Dagster Code Locations
Dagster Asset Checks
Dave & Buster's
SQLMesh
Podcast Episode
SDF
Malloy
The intro and outro music is from The Hug by The Freak Fandango Orchestra / CC BY-SASponsored By:Starburst: 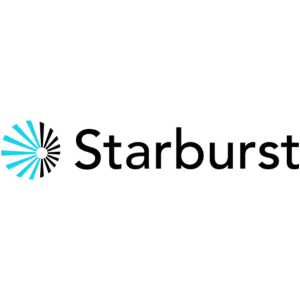
This episode is brought to you by Starburst - a data lake analytics platform for data engineers who are battling to build and scale high quality data pipelines on the data lake. Powered by Trino, Starburst runs petabyte-scale SQL analytics fast at a fraction of the cost of traditional methods, helping you meet all your data needs ranging from AI/ML workloads to data applications to complete analytics.
Trusted by the teams at Comcast and Doordash, Starburst delivers the adaptability and flexibility a lakehouse ecosystem promises, while providing a single point of access for your data and all your data governance allowing you to discover, transform, govern, and secure all in one place. Starburst does all of this on an open architecture with first-class support for Apache Iceberg, Delta Lake and Hudi, so you always maintain ownership of your data. Want to see Starburst in action? Try Starburst Galaxy today, the easiest and fastest way to get started using Trino, and get $500 of credits free. [dataengineeringpodcast.com/starburst](https://www.dataengineeringpodcast.com/starburst)Dagster: 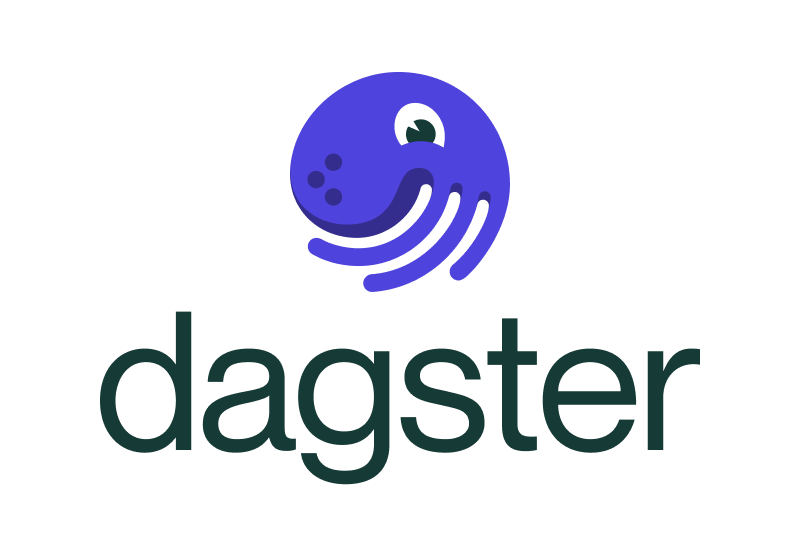
Data teams are tasked with helping organizations deliver on the premise of data, and with ML and AI maturing rapidly, expectations have never been this high. However data engineers are challenged by both technical complexity and organizational complexity, with heterogeneous technologies to adopt, multiple data disciplines converging, legacy systems to support, and costs to manage.
Dagster is an open-source orchestration solution that helps data teams reign in this complexity and build data platforms that provide unparalleled observability, and testability, all while fostering collaboration across the enterprise. With enterprise-grade hosting on Dagster Cloud, you gain even more capabilities, adding cost management, security, and CI support to further boost your teams' productivity. Go to [dagster.io](https://dagster.io/lp/dagster-cloud-trial?source=data-eng-podcast) today to get your first 30 days free!Support Data Engineering Podcast
Mar 24
55 min

Summary
A significant portion of data workflows involve storing and processing information in database engines. Validating that the information is stored and processed correctly can be complex and time-consuming, especially when the source and destination speak different dialects of SQL. In this episode Gleb Mezhanskiy, founder and CEO of Datafold, discusses the different error conditions and solutions that you need to know about to ensure the accuracy of your data.
Announcements
Hello and welcome to the Data Engineering Podcast, the show about modern data management
Dagster offers a new approach to building and running data platforms and data pipelines. It is an open-source, cloud-native orchestrator for the whole development lifecycle, with integrated lineage and observability, a declarative programming model, and best-in-class testability. Your team can get up and running in minutes thanks to Dagster Cloud, an enterprise-class hosted solution that offers serverless and hybrid deployments, enhanced security, and on-demand ephemeral test deployments. Go to dataengineeringpodcast.com/dagster today to get started. Your first 30 days are free!
Data lakes are notoriously complex. For data engineers who battle to build and scale high quality data workflows on the data lake, Starburst powers petabyte-scale SQL analytics fast, at a fraction of the cost of traditional methods, so that you can meet all your data needs ranging from AI to data applications to complete analytics. Trusted by teams of all sizes, including Comcast and Doordash, Starburst is a data lake analytics platform that delivers the adaptability and flexibility a lakehouse ecosystem promises. And Starburst does all of this on an open architecture with first-class support for Apache Iceberg, Delta Lake and Hudi, so you always maintain ownership of your data. Want to see Starburst in action? Go to dataengineeringpodcast.com/starburst and get $500 in credits to try Starburst Galaxy today, the easiest and fastest way to get started using Trino.
Join us at the top event for the global data community, Data Council Austin. From March 26-28th 2024, we'll play host to hundreds of attendees, 100 top speakers and dozens of startups that are advancing data science, engineering and AI. Data Council attendees are amazing founders, data scientists, lead engineers, CTOs, heads of data, investors and community organizers who are all working together to build the future of data and sharing their insights and learnings through deeply technical talks. As a listener to the Data Engineering Podcast you can get a special discount off regular priced and late bird tickets by using the promo code dataengpod20. Don't miss out on our only event this year! Visit dataengineeringpodcast.com/data-council and use code dataengpod20 to register today!
Your host is Tobias Macey and today I'm welcoming back Gleb Mezhanskiy to talk about how to reconcile data in database environments
Interview
Introduction
How did you get involved in the area of data management?
Can you start by outlining some of the situations where reconciling data between databases is needed?
What are examples of the error conditions that you are likely to run into when duplicating information between database engines?
When these errors do occur, what are some of the problems that they can cause?
When teams are replicating data between database engines, what are some of the common patterns for managing those flows?
How does that change between continual and one-time replication?
What are some of the steps involved in verifying the integrity of data replication between database engines?
If the source or destination isn't a traditional database engine (e.g. data lakehouse) how does that change the work involved in verifying the success of the replication?
What are the challenges of validating and reconciling data?
Sheer scale and cost of pulling data out, have to do in-place
Performance. Pushing databases to the limit, especially hard for OLTP and legacy
Cross-database compatibilty
Data types
What are the most interesting, innovative, or unexpected ways that you have seen Datafold/data-diff used in the context of cross-database validation?
What are the most interesting, unexpected, or challenging lessons that you have learned while working on Datafold?
When is Datafold/data-diff the wrong choice?
What do you have planned for the future of Datafold?
Contact Info
LinkedIn
Parting Question
From your perspective, what is the biggest gap in the tooling or technology for data management today?
Closing Announcements
Thank you for listening! Don't forget to check out our other shows. Podcast.__init__ covers the Python language, its community, and the innovative ways it is being used. The Machine Learning Podcast helps you go from idea to production with machine learning.
Visit the site to subscribe to the show, sign up for the mailing list, and read the show notes.
If you've learned something or tried out a project from the show then tell us about it! Email hosts@dataengineeringpodcast.com) with your story.
Links
Datafold
Podcast Episode
data-diff
Podcast Episode
Hive
Presto
Spark
SAP HANA
Change Data Capture
Nessie
Podcast Episode
LakeFS
Podcast Episode
Iceberg Tables
Podcast Episode
SQLGlot
Trino
GitHub Copilot
The intro and outro music is from The Hug by The Freak Fandango Orchestra / CC BY-SASponsored By:Starburst: 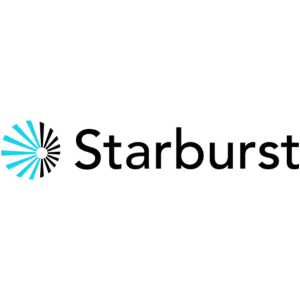
This episode is brought to you by Starburst - a data lake analytics platform for data engineers who are battling to build and scale high quality data pipelines on the data lake. Powered by Trino, Starburst runs petabyte-scale SQL analytics fast at a fraction of the cost of traditional methods, helping you meet all your data needs ranging from AI/ML workloads to data applications to complete analytics.
Trusted by the teams at Comcast and Doordash, Starburst delivers the adaptability and flexibility a lakehouse ecosystem promises, while providing a single point of access for your data and all your data governance allowing you to discover, transform, govern, and secure all in one place. Starburst does all of this on an open architecture with first-class support for Apache Iceberg, Delta Lake and Hudi, so you always maintain ownership of your data. Want to see Starburst in action? Try Starburst Galaxy today, the easiest and fastest way to get started using Trino, and get $500 of credits free. [dataengineeringpodcast.com/starburst](https://www.dataengineeringpodcast.com/starburst)Dagster: 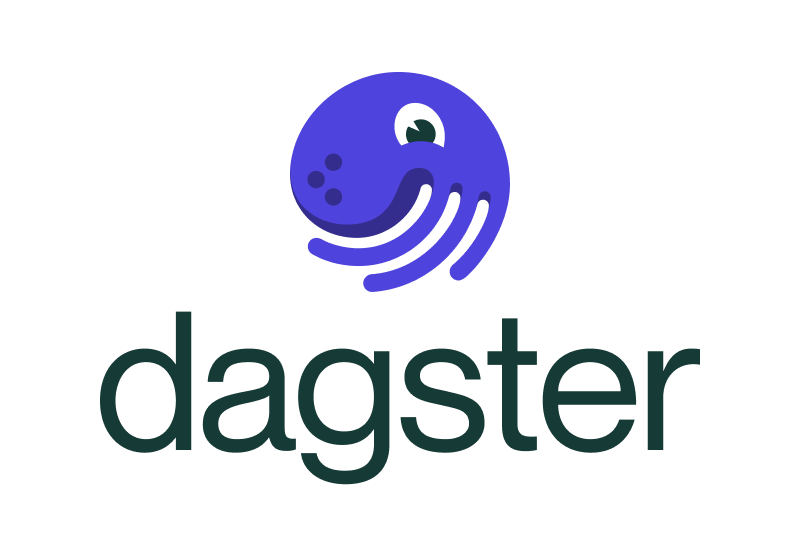
Data teams are tasked with helping organizations deliver on the premise of data, and with ML and AI maturing rapidly, expectations have never been this high. However data engineers are challenged by both technical complexity and organizational complexity, with heterogeneous technologies to adopt, multiple data disciplines converging, legacy systems to support, and costs to manage.
Dagster is an open-source orchestration solution that helps data teams reign in this complexity and build data platforms that provide unparalleled observability, and testability, all while fostering collaboration across the enterprise. With enterprise-grade hosting on Dagster Cloud, you gain even more capabilities, adding cost management, security, and CI support to further boost your teams' productivity. Go to [dagster.io](https://dagster.io/lp/dagster-cloud-trial?source=data-eng-podcast) today to get your first 30 days free!Data Council: 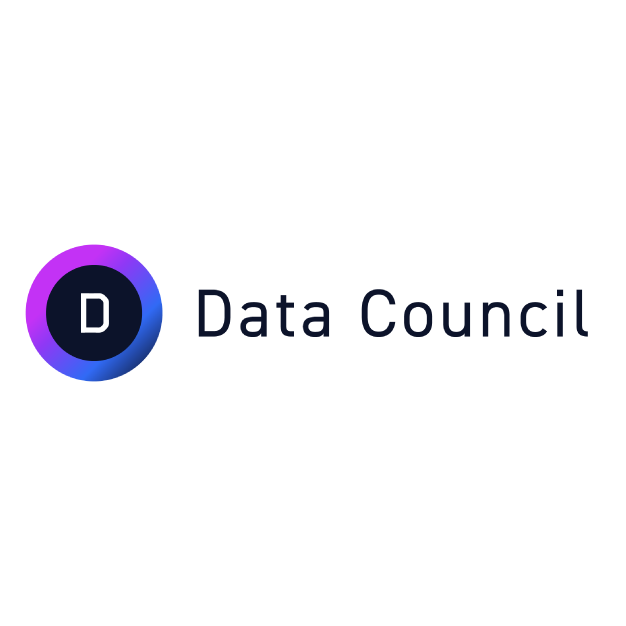
Join us at the top event for the global data community, Data Council Austin. From March 26-28th 2024, we'll play host to hundreds of attendees, 100 top speakers and dozens of startups that are advancing data science, engineering and AI. Data Council attendees are amazing founders, data scientists, lead engineers, CTOs, heads of data, investors and community organizers who are all working together to build the future of data and sharing their insights and learnings through deeply technical talks. As a listener to the Data Engineering Podcast you can get a special discount off regular priced and late bird tickets by using the promo code dataengpod20. Don't miss out on our only event this year! Visit [dataengineeringpodcast.com/data-council](https://www.dataengineeringpodcast.com/data-council) and use code **dataengpod20** to register today! Promo Code: dataengpod20Support Data Engineering Podcast
Mar 17
58 min

Summary
Data lakehouse architectures are gaining popularity due to the flexibility and cost effectiveness that they offer. The link that bridges the gap between data lake and warehouse capabilities is the catalog. The primary purpose of the catalog is to inform the query engine of what data exists and where, but the Nessie project aims to go beyond that simple utility. In this episode Alex Merced explains how the branching and merging functionality in Nessie allows you to use the same versioning semantics for your data lakehouse that you are used to from Git.
Announcements
Hello and welcome to the Data Engineering Podcast, the show about modern data management
Dagster offers a new approach to building and running data platforms and data pipelines. It is an open-source, cloud-native orchestrator for the whole development lifecycle, with integrated lineage and observability, a declarative programming model, and best-in-class testability. Your team can get up and running in minutes thanks to Dagster Cloud, an enterprise-class hosted solution that offers serverless and hybrid deployments, enhanced security, and on-demand ephemeral test deployments. Go to dataengineeringpodcast.com/dagster today to get started. Your first 30 days are free!
Data lakes are notoriously complex. For data engineers who battle to build and scale high quality data workflows on the data lake, Starburst powers petabyte-scale SQL analytics fast, at a fraction of the cost of traditional methods, so that you can meet all your data needs ranging from AI to data applications to complete analytics. Trusted by teams of all sizes, including Comcast and Doordash, Starburst is a data lake analytics platform that delivers the adaptability and flexibility a lakehouse ecosystem promises. And Starburst does all of this on an open architecture with first-class support for Apache Iceberg, Delta Lake and Hudi, so you always maintain ownership of your data. Want to see Starburst in action? Go to dataengineeringpodcast.com/starburst and get $500 in credits to try Starburst Galaxy today, the easiest and fastest way to get started using Trino.
Join us at the top event for the global data community, Data Council Austin. From March 26-28th 2024, we'll play host to hundreds of attendees, 100 top speakers and dozens of startups that are advancing data science, engineering and AI. Data Council attendees are amazing founders, data scientists, lead engineers, CTOs, heads of data, investors and community organizers who are all working together to build the future of data and sharing their insights and learnings through deeply technical talks. As a listener to the Data Engineering Podcast you can get a special discount off regular priced and late bird tickets by using the promo code dataengpod20. Don't miss out on our only event this year! Visit dataengineeringpodcast.com/data-council and use code dataengpod20 to register today!
Your host is Tobias Macey and today I'm interviewing Alex Merced, developer advocate at Dremio and co-author of the upcoming book from O'reilly, "Apache Iceberg, The definitive Guide", about Nessie, a git-like versioned catalog for data lakes using Apache Iceberg
Interview
Introduction
How did you get involved in the area of data management?
Can you describe what Nessie is and the story behind it?
What are the core problems/complexities that Nessie is designed to solve?
The closest analogue to Nessie that I've seen in the ecosystem is LakeFS. What are the features that would lead someone to choose one or the other for a given use case?
Why would someone choose Nessie over native table-level branching in the Apache Iceberg spec?
How do the versioning capabilities compare to/augment the data versioning in Iceberg?
What are some of the sources of, and challenges in resolving, merge conflicts between table branches?
Can you describe the architecture of Nessie?
How have the design and goals of the project changed since it was first created?
What is involved in integrating Nessie into a given data stack?
For cases where a given query/compute engine doesn't natively support Nessie, what are the options for using it effectively?
How does the inclusion of Nessie in a data lake influence the overall workflow of developing/deploying/evolving processing flows?
What are the most interesting, innovative, or unexpected ways that you have seen Nessie used?
What are the most interesting, unexpected, or challenging lessons that you have learned while working with Nessie?
When is Nessie the wrong choice?
What have you heard is planned for the future of Nessie?
Contact Info
LinkedIn
Twitter
Alex's Article on Dremio's Blog
Alex's Substack
Parting Question
From your perspective, what is the biggest gap in the tooling or technology for data management today?
Closing Announcements
Thank you for listening! Don't forget to check out our other shows. Podcast.__init__ covers the Python language, its community, and the innovative ways it is being used. The Machine Learning Podcast helps you go from idea to production with machine learning.
Visit the site to subscribe to the show, sign up for the mailing list, and read the show notes.
If you've learned something or tried out a project from the show then tell us about it! Email hosts@dataengineeringpodcast.com) with your story.
Links
Project Nessie
Article: What is Nessie, Catalog Versioning and Git-for-Data?
Article: What is Lakehouse Management?: Git-for-Data, Automated Apache Iceberg Table Maintenance and more
Free Early Release Copy of "Apache Iceberg: The Definitive Guide"
Iceberg
Podcast Episode
Arrow
Podcast Episode
Data Lakehouse
LakeFS
Podcast Episode
AWS Glue
Tabular
Podcast Episode
Trino
Presto
Dremio
Podcast Episode
RocksDB
Delta Lake
Podcast Episode
Hive Metastore
PyIceberg
Optimistic Concurrency Control
The intro and outro music is from The Hug by The Freak Fandango Orchestra / CC BY-SASponsored By:Starburst: 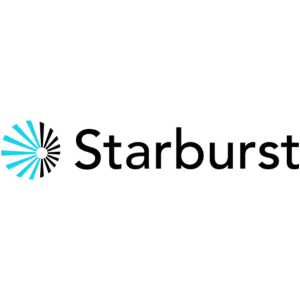
This episode is brought to you by Starburst - a data lake analytics platform for data engineers who are battling to build and scale high quality data pipelines on the data lake. Powered by Trino, Starburst runs petabyte-scale SQL analytics fast at a fraction of the cost of traditional methods, helping you meet all your data needs ranging from AI/ML workloads to data applications to complete analytics.
Trusted by the teams at Comcast and Doordash, Starburst delivers the adaptability and flexibility a lakehouse ecosystem promises, while providing a single point of access for your data and all your data governance allowing you to discover, transform, govern, and secure all in one place. Starburst does all of this on an open architecture with first-class support for Apache Iceberg, Delta Lake and Hudi, so you always maintain ownership of your data. Want to see Starburst in action? Try Starburst Galaxy today, the easiest and fastest way to get started using Trino, and get $500 of credits free. [dataengineeringpodcast.com/starburst](https://www.dataengineeringpodcast.com/starburst)Data Council: 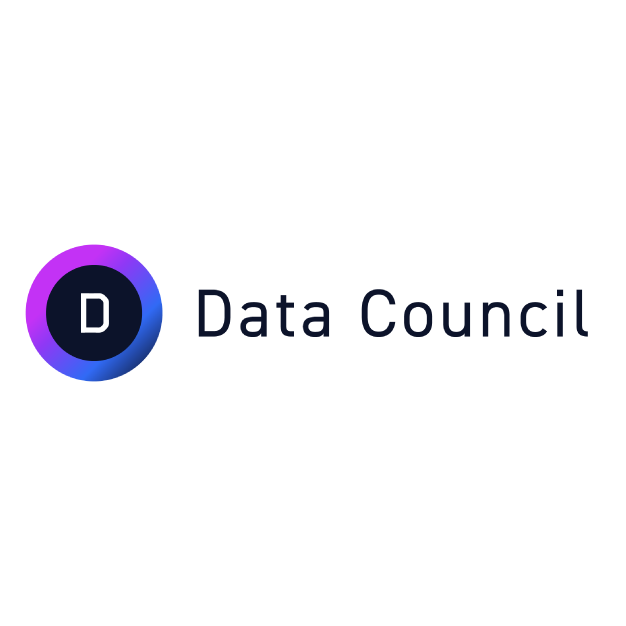
Join us at the top event for the global data community, Data Council Austin. From March 26-28th 2024, we'll play host to hundreds of attendees, 100 top speakers and dozens of startups that are advancing data science, engineering and AI. Data Council attendees are amazing founders, data scientists, lead engineers, CTOs, heads of data, investors and community organizers who are all working together to build the future of data and sharing their insights and learnings through deeply technical talks. As a listener to the Data Engineering Podcast you can get a special discount off regular priced and late bird tickets by using the promo code dataengpod20. Don't miss out on our only event this year! Visit [dataengineeringpodcast.com/data-council](https://www.dataengineeringpodcast.com/data-council) and use code **dataengpod20** to register today! Promo Code: dataengpod20Dagster: 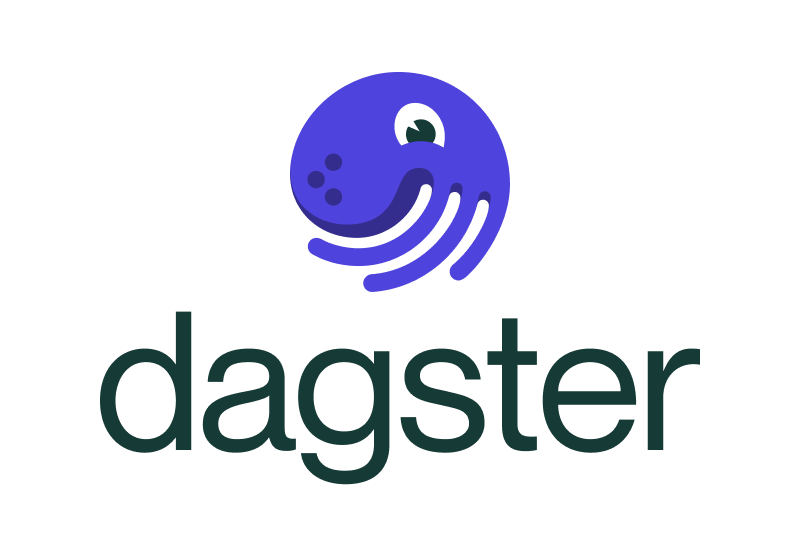
Data teams are tasked with helping organizations deliver on the premise of data, and with ML and AI maturing rapidly, expectations have never been this high. However data engineers are challenged by both technical complexity and organizational complexity, with heterogeneous technologies to adopt, multiple data disciplines converging, legacy systems to support, and costs to manage.
Dagster is an open-source orchestration solution that helps data teams reign in this complexity and build data platforms that provide unparalleled observability, and testability, all while fostering collaboration across the enterprise. With enterprise-grade hosting on Dagster Cloud, you gain even more capabilities, adding cost management, security, and CI support to further boost your teams' productivity. Go to [dagster.io](https://dagster.io/lp/dagster-cloud-trial?source=data-eng-podcast) today to get your first 30 days free!Support Data Engineering Podcast
Mar 10
40 min

Summary
Artificial intelligence technologies promise to revolutionize business and produce new sources of value. In order to make those promises a reality there is a substantial amount of strategy and investment required. Colleen Tartow has worked across all stages of the data lifecycle, and in this episode she shares her hard-earned wisdom about how to conduct an AI program for your organization.
Announcements
Hello and welcome to the Data Engineering Podcast, the show about modern data management
Dagster offers a new approach to building and running data platforms and data pipelines. It is an open-source, cloud-native orchestrator for the whole development lifecycle, with integrated lineage and observability, a declarative programming model, and best-in-class testability. Your team can get up and running in minutes thanks to Dagster Cloud, an enterprise-class hosted solution that offers serverless and hybrid deployments, enhanced security, and on-demand ephemeral test deployments. Go to dataengineeringpodcast.com/dagster today to get started. Your first 30 days are free!
Data lakes are notoriously complex. For data engineers who battle to build and scale high quality data workflows on the data lake, Starburst powers petabyte-scale SQL analytics fast, at a fraction of the cost of traditional methods, so that you can meet all your data needs ranging from AI to data applications to complete analytics. Trusted by teams of all sizes, including Comcast and Doordash, Starburst is a data lake analytics platform that delivers the adaptability and flexibility a lakehouse ecosystem promises. And Starburst does all of this on an open architecture with first-class support for Apache Iceberg, Delta Lake and Hudi, so you always maintain ownership of your data. Want to see Starburst in action? Go to dataengineeringpodcast.com/starburst and get $500 in credits to try Starburst Galaxy today, the easiest and fastest way to get started using Trino.
Join us at the top event for the global data community, Data Council Austin. From March 26-28th 2024, we'll play host to hundreds of attendees, 100 top speakers and dozens of startups that are advancing data science, engineering and AI. Data Council attendees are amazing founders, data scientists, lead engineers, CTOs, heads of data, investors and community organizers who are all working together to build the future of data and sharing their insights and learnings through deeply technical talks. As a listener to the Data Engineering Podcast you can get a special discount off regular priced and late bird tickets by using the promo code dataengpod20. Don't miss out on our only event this year! Visit dataengineeringpodcast.com/data-council and use code dataengpod20 to register today!
Your host is Tobias Macey and today I'm interviewing Colleen Tartow about the questions to answer before and during the development of an AI program
Interview
Introduction
How did you get involved in the area of data management?
When you say "AI Program", what are the organizational, technical, and strategic elements that it encompasses?
How does the idea of an "AI Program" differ from an "AI Product"?
What are some of the signals to watch for that indicate an objective for which AI is not a reasonable solution?
Who needs to be involved in the process of defining and developing that program?
What are the skills and systems that need to be in place to effectively execute on an AI program?
"AI" has grown to be an even more overloaded term than it already was. What are some of the useful clarifying/scoping questions to address when deciding the path to deployment for different definitions of "AI"?
Organizations can easily fall into the trap of green-lighting an AI project before they have done the work of ensuring they have the necessary data and the ability to process it. What are the steps to take to build confidence in the availability of the data?
Even if you are sure that you can get the data, what are the implementation pitfalls that teams should be wary of while building out the data flows for powering the AI system?
What are the key considerations for powering AI applications that are substantially different from analytical applications?
The ecosystem for ML/AI is a rapidly moving target. What are the foundational/fundamental principles that you need to design around to allow for future flexibility?
What are the most interesting, innovative, or unexpected ways that you have seen AI programs implemented?
What are the most interesting, unexpected, or challenging lessons that you have learned while working on powering AI systems?
When is AI the wrong choice?
What do you have planned for the future of your work at VAST Data?
Contact Info
LinkedIn
Parting Question
From your perspective, what is the biggest gap in the tooling or technology for data management today?
Closing Announcements
Thank you for listening! Don't forget to check out our other shows. Podcast.__init__ covers the Python language, its community, and the innovative ways it is being used. The Machine Learning Podcast helps you go from idea to production with machine learning.
Visit the site to subscribe to the show, sign up for the mailing list, and read the show notes.
If you've learned something or tried out a project from the show then tell us about it! Email hosts@dataengineeringpodcast.com) with your story.
Links
VAST Data
Colleen's Previous Appearance
Linear Regression
CoreWeave
Lambda Labs
MAD Landscape
Podcast Episode
ML Episode
The intro and outro music is from The Hug by The Freak Fandango Orchestra / CC BY-SASponsored By:Dagster: 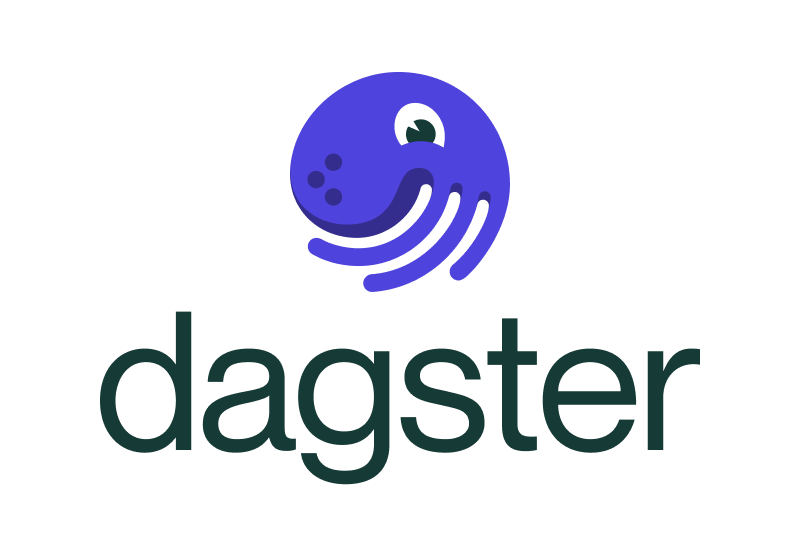
Data teams are tasked with helping organizations deliver on the premise of data, and with ML and AI maturing rapidly, expectations have never been this high. However data engineers are challenged by both technical complexity and organizational complexity, with heterogeneous technologies to adopt, multiple data disciplines converging, legacy systems to support, and costs to manage.
Dagster is an open-source orchestration solution that helps data teams reign in this complexity and build data platforms that provide unparalleled observability, and testability, all while fostering collaboration across the enterprise. With enterprise-grade hosting on Dagster Cloud, you gain even more capabilities, adding cost management, security, and CI support to further boost your teams' productivity. Go to [dagster.io](https://dagster.io/lp/dagster-cloud-trial?source=data-eng-podcast) today to get your first 30 days free!Data Council: 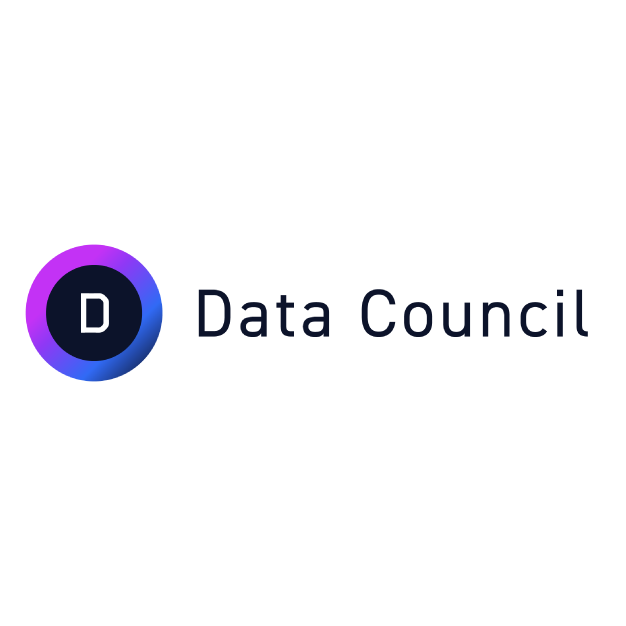
Join us at the top event for the global data community, Data Council Austin. From March 26-28th 2024, we'll play host to hundreds of attendees, 100 top speakers and dozens of startups that are advancing data science, engineering and AI. Data Council attendees are amazing founders, data scientists, lead engineers, CTOs, heads of data, investors and community organizers who are all working together to build the future of data and sharing their insights and learnings through deeply technical talks. As a listener to the Data Engineering Podcast you can get a special discount off regular priced and late bird tickets by using the promo code dataengpod20. Don't miss out on our only event this year! Visit [dataengineeringpodcast.com/data-council](https://www.dataengineeringpodcast.com/data-council) and use code **dataengpod20** to register today! Promo Code: dataengpod20Starburst: 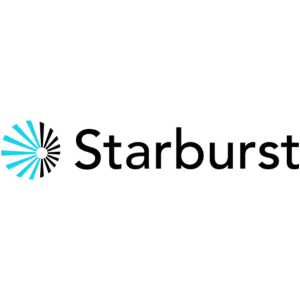
This episode is brought to you by Starburst - a data lake analytics platform for data engineers who are battling to build and scale high quality data pipelines on the data lake. Powered by Trino, Starburst runs petabyte-scale SQL analytics fast at a fraction of the cost of traditional methods, helping you meet all your data needs ranging from AI/ML workloads to data applications to complete analytics.
Trusted by the teams at Comcast and Doordash, Starburst delivers the adaptability and flexibility a lakehouse ecosystem promises, while providing a single point of access for your data and all your data governance allowing you to discover, transform, govern, and secure all in one place. Starburst does all of this on an open architecture with first-class support for Apache Iceberg, Delta Lake and Hudi, so you always maintain ownership of your data. Want to see Starburst in action? Try Starburst Galaxy today, the easiest and fastest way to get started using Trino, and get $500 of credits free. [dataengineeringpodcast.com/starburst](https://www.dataengineeringpodcast.com/starburst)Support Data Engineering Podcast
Mar 3
46 min

Summary
Building a database engine requires a substantial amount of engineering effort and time investment. Over the decades of research and development into building these software systems there are a number of common components that are shared across implementations. When Paul Dix decided to re-write the InfluxDB engine he found the Apache Arrow ecosystem ready and waiting with useful building blocks to accelerate the process. In this episode he explains how he used the combination of Apache Arrow, Flight, Datafusion, and Parquet to lay the foundation of the newest version of his time-series database.
Announcements
Hello and welcome to the Data Engineering Podcast, the show about modern data management
Dagster offers a new approach to building and running data platforms and data pipelines. It is an open-source, cloud-native orchestrator for the whole development lifecycle, with integrated lineage and observability, a declarative programming model, and best-in-class testability. Your team can get up and running in minutes thanks to Dagster Cloud, an enterprise-class hosted solution that offers serverless and hybrid deployments, enhanced security, and on-demand ephemeral test deployments. Go to dataengineeringpodcast.com/dagster today to get started. Your first 30 days are free!
Data lakes are notoriously complex. For data engineers who battle to build and scale high quality data workflows on the data lake, Starburst powers petabyte-scale SQL analytics fast, at a fraction of the cost of traditional methods, so that you can meet all your data needs ranging from AI to data applications to complete analytics. Trusted by teams of all sizes, including Comcast and Doordash, Starburst is a data lake analytics platform that delivers the adaptability and flexibility a lakehouse ecosystem promises. And Starburst does all of this on an open architecture with first-class support for Apache Iceberg, Delta Lake and Hudi, so you always maintain ownership of your data. Want to see Starburst in action? Go to dataengineeringpodcast.com/starburst and get $500 in credits to try Starburst Galaxy today, the easiest and fastest way to get started using Trino.
Join us at the top event for the global data community, Data Council Austin. From March 26-28th 2024, we'll play host to hundreds of attendees, 100 top speakers and dozens of startups that are advancing data science, engineering and AI. Data Council attendees are amazing founders, data scientists, lead engineers, CTOs, heads of data, investors and community organizers who are all working together to build the future of data and sharing their insights and learnings through deeply technical talks. As a listener to the Data Engineering Podcast you can get a special discount off regular priced and late bird tickets by using the promo code dataengpod20. Don't miss out on our only event this year! Visit dataengineeringpodcast.com/data-council and use code dataengpod20 to register today!
Your host is Tobias Macey and today I'm interviewing Paul Dix about his investment in the Apache Arrow ecosystem and how it led him to create the latest PFAD in database design
Interview
Introduction
How did you get involved in the area of data management?
Can you start by describing the FDAP stack and how the components combine to provide a foundational architecture for database engines?
This was the core of your recent re-write of the InfluxDB engine. What were the design goals and constraints that led you to this architecture?
Each of the architectural components are well engineered for their particular scope. What is the engineering work that is involved in building a cohesive platform from those components?
One of the major benefits of using open source components is the network effect of ecosystem integrations. That can also be a risk when the community vision for the project doesn't align with your own goals. How have you worked to mitigate that risk in your specific platform?
Can you describe the operational/architectural aspects of building a full data engine on top of the FDAP stack?
What are the elements of the overall product/user experience that you had to build to create a cohesive platform?
What are some of the other tools/technologies that can benefit from some or all of the pieces of the FDAP stack?
What are the pieces of the Arrow ecosystem that are still immature or need further investment from the community?
What are the most interesting, innovative, or unexpected ways that you have seen parts or all of the FDAP stack used?
What are the most interesting, unexpected, or challenging lessons that you have learned while working on/with the FDAP stack?
When is the FDAP stack the wrong choice?
What do you have planned for the future of the InfluxDB IOx engine and the FDAP stack?
Contact Info
LinkedIn
pauldix on GitHub
Parting Question
From your perspective, what is the biggest gap in the tooling or technology for data management today?
Closing Announcements
Thank you for listening! Don't forget to check out our other shows. Podcast.__init__ covers the Python language, its community, and the innovative ways it is being used. The Machine Learning Podcast helps you go from idea to production with machine learning.
Visit the site to subscribe to the show, sign up for the mailing list, and read the show notes.
If you've learned something or tried out a project from the show then tell us about it! Email hosts@dataengineeringpodcast.com) with your story.
Links
FDAP Stack Blog Post
Apache Arrow
DataFusion
Arrow Flight
Apache Parquet
InfluxDB
Influx Data
Podcast Episode
Rust Language
DuckDB
ClickHouse
Voltron Data
Podcast Episode
Velox
Iceberg
Podcast Episode
Trino
ODBC == Open DataBase Connectivity
GeoParquet
ORC == Optimized Row Columnar
Avro
Protocol Buffers
gRPC
The intro and outro music is from The Hug by The Freak Fandango Orchestra / CC BY-SASponsored By:Starburst: 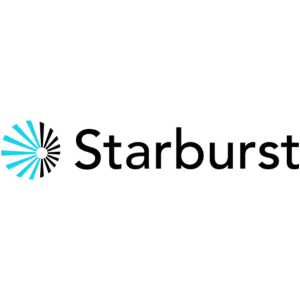
This episode is brought to you by Starburst - a data lake analytics platform for data engineers who are battling to build and scale high quality data pipelines on the data lake. Powered by Trino, Starburst runs petabyte-scale SQL analytics fast at a fraction of the cost of traditional methods, helping you meet all your data needs ranging from AI/ML workloads to data applications to complete analytics.
Trusted by the teams at Comcast and Doordash, Starburst delivers the adaptability and flexibility a lakehouse ecosystem promises, while providing a single point of access for your data and all your data governance allowing you to discover, transform, govern, and secure all in one place. Starburst does all of this on an open architecture with first-class support for Apache Iceberg, Delta Lake and Hudi, so you always maintain ownership of your data. Want to see Starburst in action? Try Starburst Galaxy today, the easiest and fastest way to get started using Trino, and get $500 of credits free. [dataengineeringpodcast.com/starburst](https://www.dataengineeringpodcast.com/starburst)Data Council: 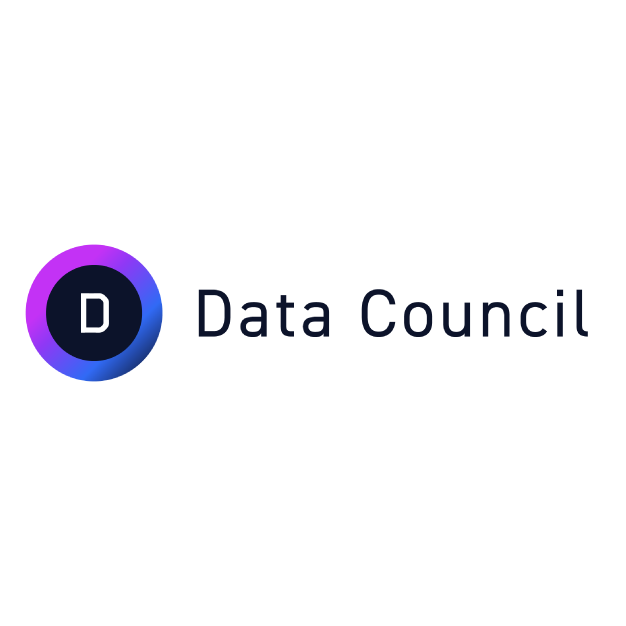
Join us at the top event for the global data community, Data Council Austin. From March 26-28th 2024, we'll play host to hundreds of attendees, 100 top speakers and dozens of startups that are advancing data science, engineering and AI. Data Council attendees are amazing founders, data scientists, lead engineers, CTOs, heads of data, investors and community organizers who are all working together to build the future of data and sharing their insights and learnings through deeply technical talks. As a listener to the Data Engineering Podcast you can get a special discount off regular priced and late bird tickets by using the promo code dataengpod20. Don't miss out on our only event this year! Visit [dataengineeringpodcast.com/data-council](https://www.dataengineeringpodcast.com/data-council) and use code **dataengpod20** to register today! Promo Code: dataengpod20Dagster: 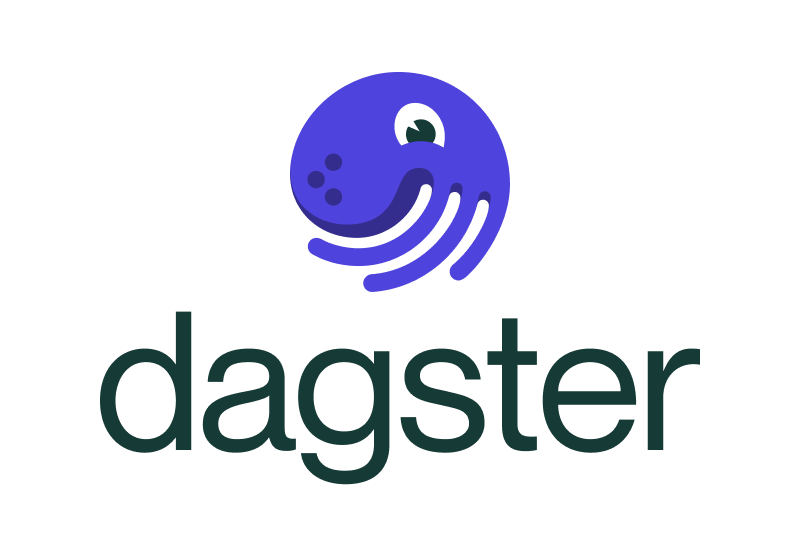
Data teams are tasked with helping organizations deliver on the premise of data, and with ML and AI maturing rapidly, expectations have never been this high. However data engineers are challenged by both technical complexity and organizational complexity, with heterogeneous technologies to adopt, multiple data disciplines converging, legacy systems to support, and costs to manage.
Dagster is an open-source orchestration solution that helps data teams reign in this complexity and build data platforms that provide unparalleled observability, and testability, all while fostering collaboration across the enterprise. With enterprise-grade hosting on Dagster Cloud, you gain even more capabilities, adding cost management, security, and CI support to further boost your teams' productivity. Go to [dagster.io](https://dagster.io/lp/dagster-cloud-trial?source=data-eng-podcast) today to get your first 30 days free!Support Data Engineering Podcast
Feb 25
56 min

Summary
A data lakehouse is intended to combine the benefits of data lakes (cost effective, scalable storage and compute) and data warehouses (user friendly SQL interface). Multiple open source projects and vendors have been working together to make this vision a reality. In this episode Dain Sundstrom, CTO of Starburst, explains how the combination of the Trino query engine and the Iceberg table format offer the ease of use and execution speed of data warehouses with the infinite storage and scalability of data lakes.
Announcements
Hello and welcome to the Data Engineering Podcast, the show about modern data management
Dagster offers a new approach to building and running data platforms and data pipelines. It is an open-source, cloud-native orchestrator for the whole development lifecycle, with integrated lineage and observability, a declarative programming model, and best-in-class testability. Your team can get up and running in minutes thanks to Dagster Cloud, an enterprise-class hosted solution that offers serverless and hybrid deployments, enhanced security, and on-demand ephemeral test deployments. Go to dataengineeringpodcast.com/dagster today to get started. Your first 30 days are free!
Data lakes are notoriously complex. For data engineers who battle to build and scale high quality data workflows on the data lake, Starburst powers petabyte-scale SQL analytics fast, at a fraction of the cost of traditional methods, so that you can meet all your data needs ranging from AI to data applications to complete analytics. Trusted by teams of all sizes, including Comcast and Doordash, Starburst is a data lake analytics platform that delivers the adaptability and flexibility a lakehouse ecosystem promises. And Starburst does all of this on an open architecture with first-class support for Apache Iceberg, Delta Lake and Hudi, so you always maintain ownership of your data. Want to see Starburst in action? Go to dataengineeringpodcast.com/starburst and get $500 in credits to try Starburst Galaxy today, the easiest and fastest way to get started using Trino.
Join in with the event for the global data community, Data Council Austin. From March 26th-28th 2024, they'll play host to hundreds of attendees, 100 top speakers, and dozens of startups that are advancing data science, engineering and AI. Data Council attendees are amazing founders, data scientists, lead engineers, CTOs, heads of data, investors and community organizers who are all working togethr to build the future of data. As a listener to the Data Engineering Podcast you can get a special discount of 20% off your ticket by using the promo code dataengpod20. Don't miss out on their only event this year! Visit: dataengineeringpodcast.com/data-council today.
Your host is Tobias Macey and today I'm interviewing Dain Sundstrom about building a data lakehouse with Trino and Iceberg
Interview
Introduction
How did you get involved in the area of data management?
To start, can you share your definition of what constitutes a "Data Lakehouse"?
What are the technical/architectural/UX challenges that have hindered the progression of lakehouses?
What are the notable advancements in recent months/years that make them a more viable platform choice?
There are multiple tools and vendors that have adopted the "data lakehouse" terminology. What are the benefits offered by the combination of Trino and Iceberg?
What are the key points of comparison for that combination in relation to other possible selections?
What are the pain points that are still prevalent in lakehouse architectures as compared to warehouse or vertically integrated systems?
What progress is being made (within or across the ecosystem) to address those sharp edges?
For someone who is interested in building a data lakehouse with Trino and Iceberg, how does that influence their selection of other platform elements?
What are the differences in terms of pipeline design/access and usage patterns when using a Trino/Iceberg lakehouse as compared to other popular warehouse/lakehouse structures?
What are the most interesting, innovative, or unexpected ways that you have seen Trino lakehouses used?
What are the most interesting, unexpected, or challenging lessons that you have learned while working on the data lakehouse ecosystem?
When is a lakehouse the wrong choice?
What do you have planned for the future of Trino/Starburst?
Contact Info
LinkedIn
dain on GitHub
Parting Question
From your perspective, what is the biggest gap in the tooling or technology for data management today?
Closing Announcements
Thank you for listening! Don't forget to check out our other shows. Podcast.__init__ covers the Python language, its community, and the innovative ways it is being used. The Machine Learning Podcast helps you go from idea to production with machine learning.
Visit the site to subscribe to the show, sign up for the mailing list, and read the show notes.
If you've learned something or tried out a project from the show then tell us about it! Email hosts@dataengineeringpodcast.com) with your story.
Links
Trino
Starburst
Presto
JBoss
Java EE
HDFS
S3
GCS == Google Cloud Storage
Hive
Hive ACID
Apache Ranger
OPA == Open Policy Agent
Oso
AWS Lakeformation
Tabular
Iceberg
Podcast Episode
Delta Lake
Podcast Episode
Debezium
Podcast Episode
Materialized View
Clickhouse
Druid
Hudi
Podcast Episode
The intro and outro music is from The Hug by The Freak Fandango Orchestra / CC BY-SASponsored By:Data Council: 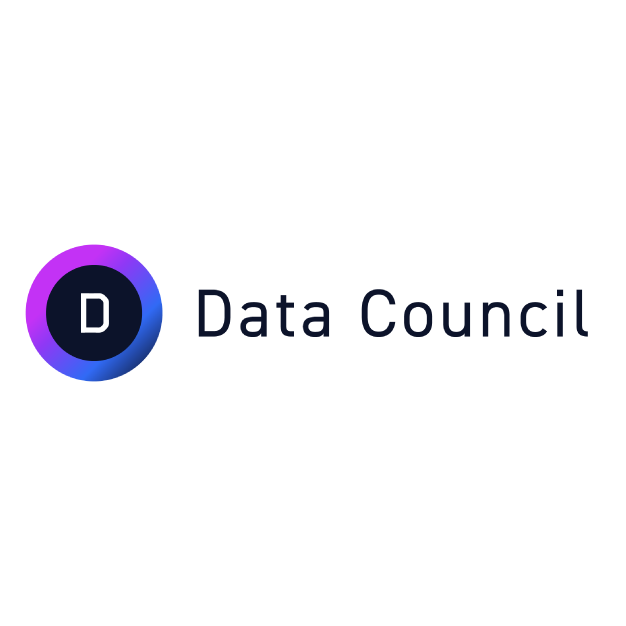
Join us at the top event for the global data community, Data Council Austin. From March 26-28th 2024, we'll play host to hundreds of attendees, 100 top speakers and dozens of startups that are advancing data science, engineering and AI. Data Council attendees are amazing founders, data scientists, lead engineers, CTOs, heads of data, investors and community organizers who are all working together to build the future of data and sharing their insights and learnings through deeply technical talks. As a listener to the Data Engineering Podcast you can get a special discount off regular priced and late bird tickets by using the promo code dataengpod20. Don't miss out on our only event this year! Visit [dataengineeringpodcast.com/data-council](https://www.dataengineeringpodcast.com/data-council) and use code **dataengpod20** to register today! Promo Code: dataengpod20Starburst: 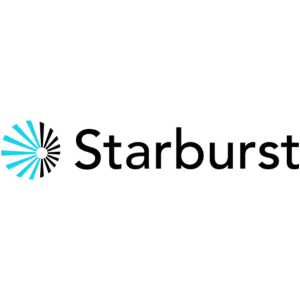
This episode is brought to you by Starburst - a data lake analytics platform for data engineers who are battling to build and scale high quality data pipelines on the data lake. Powered by Trino, Starburst runs petabyte-scale SQL analytics fast at a fraction of the cost of traditional methods, helping you meet all your data needs ranging from AI/ML workloads to data applications to complete analytics.
Trusted by the teams at Comcast and Doordash, Starburst delivers the adaptability and flexibility a lakehouse ecosystem promises, while providing a single point of access for your data and all your data governance allowing you to discover, transform, govern, and secure all in one place. Starburst does all of this on an open architecture with first-class support for Apache Iceberg, Delta Lake and Hudi, so you always maintain ownership of your data. Want to see Starburst in action? Try Starburst Galaxy today, the easiest and fastest way to get started using Trino, and get $500 of credits free. [dataengineeringpodcast.com/starburst](https://www.dataengineeringpodcast.com/starburst)Dagster: 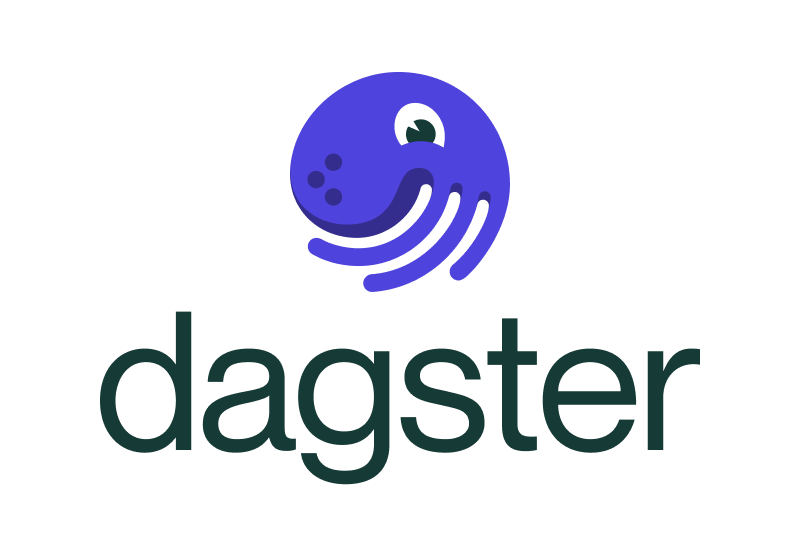
Data teams are tasked with helping organizations deliver on the premise of data, and with ML and AI maturing rapidly, expectations have never been this high. However data engineers are challenged by both technical complexity and organizational complexity, with heterogeneous technologies to adopt, multiple data disciplines converging, legacy systems to support, and costs to manage.
Dagster is an open-source orchestration solution that helps data teams reign in this complexity and build data platforms that provide unparalleled observability, and testability, all while fostering collaboration across the enterprise. With enterprise-grade hosting on Dagster Cloud, you gain even more capabilities, adding cost management, security, and CI support to further boost your teams' productivity. Go to [dagster.io](https://dagster.io/lp/dagster-cloud-trial?source=data-eng-podcast) today to get your first 30 days free!Support Data Engineering Podcast
Feb 18
58 min
Load more